The 12 deadly sins in the utilization of process data!
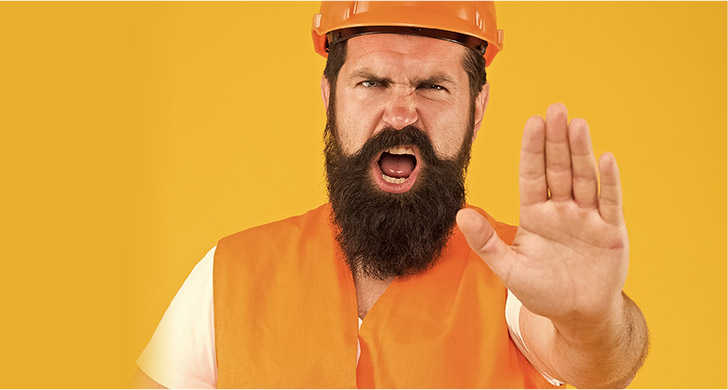
We all want to make the most from our process data, but occasionally we fail to do so. Check out our list of the 12 deadly sins in the utilization of process data!
Are any of these familiar?
1. Lack of curiosity
Is blind faith in old presumptions about process behavior persisting in your team, or has a large amount of data turned into a scary boogeyman?
Be bold! If you do not or dare not search for anything, you will not find anything either. A curious and bold approach is a must!
2. You can prove anything with facts…
Even Homer Simpson knows that you can prove anything with facts. The same goes for process analysis. If the outcome is already decided and you only need data to prove it, you will surely find ways to do that. A sufficiently large mass of data is like a soft piece of modeling wax that you can mold into the desired form.
Be honest and open when you process data.
Analyses are often jumped into with a good attitude and enthusiasm, but setbacks are encountered right off the bat in the early stages of the process. The reason is often all too human: brimmed with enthusiasm, people fail to thoroughly consider how the problem should be approached and with what kind of expectations. This often results in frustration.
3. What exactly is your problem?
Data is eagerly analyzed without taking the time needed to think about a clearly defined goal. You will surely learn new things about the process this way as well, but the results tend to remain meagre.
Ask right questions. To maximize the usefulness of the results, pause for a while before starting to handle the data and think which question, above all others, you wish to find an answer to. The better the question is delineated, the better your prerequisites for success.
Unlock the power of Wedge for industrial data analytics
4. A strong belief is haunting
Unlike in point #2 above, you are seriously analyzing the data and eager to find answers. Nevertheless, the team’s tacit assumptions may subtly direct the analysis process toward an old conclusion that is presumed to be good.
Dare to experiment with new methods, incorporate new variables into the analysis and keep the set of measurements being studied sufficiently broad. This way, you will ensure that there is room left for new conclusions.
5. There is a magic bullet
A complex set of issues only becomes simple in a populist’s speech. Don’t imagine that data analysis would make any exception to this rule! Even the prettiest data set won’t give you a straightforward answer to everything (or maybe it will, but the answer will probably be useless) – not even with Wedge.
In reality, you will learn more with each round of analysis and approach the solution step by step. Your original question will often be refined along the way and you will eventually get the correct answer, but to the question you have rephrased.
6. Someone else will take care of it
Busy, busy, busy, you are so busy with pushing your moped that there is no time to hop onto the saddle and start the engine. But the world is full of consultants – why not just throw them the data and let them analyze it? That would indeed suit the consultants! On top of it all, you’ll get a hefty bill and a future as a cash cow that can always be billed when you’ve got problems.
Surely you do not want to outsource the understanding of process behavior and process competence? So do not outsource the analysis of process data. It is an important part of your core competence.
7. Who will turn shit into diamonds?
If the data being analyzed is no good, the results will be crap as well. Process data as such is nearly always worthless, but all the same, it is the best raw material that is available. After all, even diamonds are made of carbon.
Use right tool. Data pre-processing is one of the most important steps in the analysis. How do you clean up bad data, compensate for process delays and focus your analysis solely on the desired end product? A tool will help you with this.
If you make compromises with this part, you can forget about the entire analysis. Accept the quality of the raw material and behave in a way that will get you a diamond-like end result.
8. You try to cut corners
In the absence of means, it is tempting to cut corners. Deadlines are pushing and the work proceeds slowly.
Maybe you should choose a limited data set and completely ignore the lab data? You just clean up the data superficially and ignore the delays. You make quick and narrow analyses. You accept that the understanding of causal relationships remains very feeble.
Sorry, but quick and dirty is not enough. Invest in proper tools that allow you to do better and more versatile analysis, and you will really save time.
9. Agonizing death by drowning
There is a lot of data. Tens of thousands of measurements made every second and over several years. The ambitions are high indeed. The goal is clear.
You want to thoroughly clean up your data and take a sufficiently large set of measurements into account in your analysis. You iterate, add measurements to the analysis, refine the angle of incidence, and sweat. The data masses begin to submerge you, and at the same time you notice that the measurement data is not all-inclusive. Nightmares invade the daydreaming.
Use powerful tools to help you dig up the essential data. Analyze the phenomena from multiple perspectives and change direction quickly if needed to ensure that you stay on the right track on your way to your goal.
10. Simple tools to address complex problems
The quality measurement of the end product bounces up and down. The eye detects periodic variation, but you cannot quantify or detect the root cause.
2D profile data looks bad, but how do you mirror 2D data against the other process data?
Make complex simple. What if the phenomenon you are investigating really is complex? Sometimes you need to take recourse to more robust tools if you want results.
11. Lack of process understanding
Data, data, data. Still, data does not tell you everything, and no one anywhere will ever be able to measure everything. Even at its best, a tool can only suggest candidates for root causes or effects. Machine-learning systems are easy to market as omnipotent, but without the engineer’s process competence, all data analysis is worthless.
The value is in the conclusions, not in the analysis process!
12. Lack of data analysis competence
Even the best process expert will be stumped if he is urged to analyze data without sufficient data processing competence and user-friendly tools.
The better the tools, the fewer magical powers it will take to succeed in analyses! For this reason, we have developed Wedge into a tool that does not require the user to have the skills of a data analyst to obtain results.
Thank you for your interest!
Want more insights?
Our sales team is full of data analysis experts ready to help you. Learn more about Wedge industrial data analytics tool.