Harnessing industrial data: Turning fragmented data into competitive edge
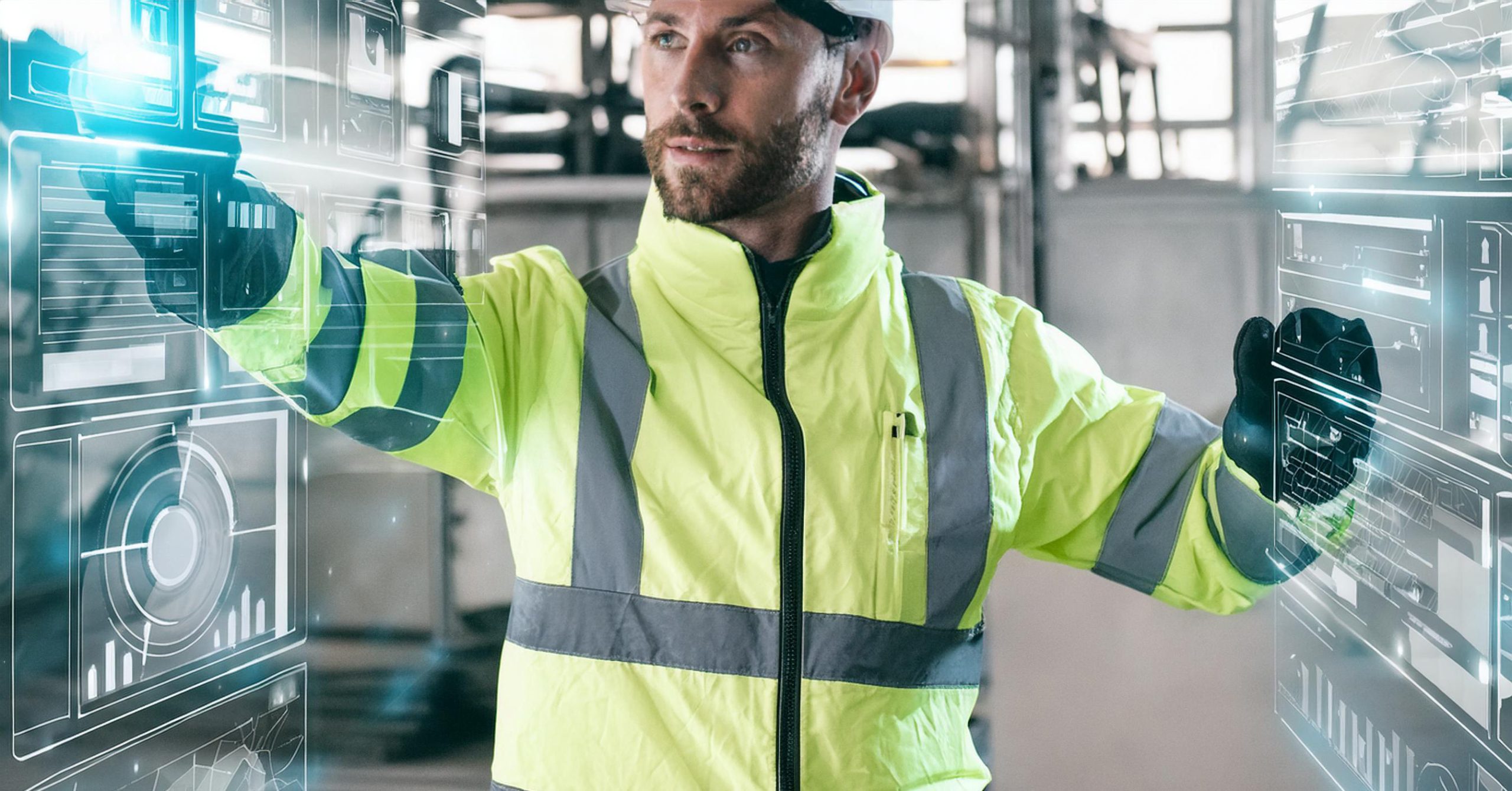
The amount of data collected from industrial processes is increasing at an accelerating speed. Modern computer systems allow data to be stored over long periods of time and at a much faster sampling rate than ever before. This raises an important question: What do we do with all this data?
BtoC companies have been successfully collecting and analyzing large and complex customer transaction data for several years. This ‘big data approach’ aims to give them a competitive edge over their rivals. Industrial BtoB companies are not at the same level yet, but the ever-increasing competition forces them to fine-tune their manufacturing processes. Companies are forced to produce better quality in larger amounts and, at the same time, reduce energy consumption and raw material costs.
These problems can be solved by allowing employees to improve manufacturing processes based on measured time series data.
By applying efficient data handling methods and tools, industrial companies can unearth the untapped potential in their existing data, leading to improved process diagnostics and a competitive edge.
Challenges of utilizing fragmented data
During the last decade, digital information, process control and business execution systems have become standard applications in the pulp and paper industry. At the same time, the amounts of data collected and saved have increased significantly, and this trend will continue in the future.
Mills’ systems are typically focused on either a limited process area (e.g., raw material handling, stock preparation, reel section) or a limited data type (e.g., control loop data, process data, quality data and cost data).
Today, huge amounts of data are collected, but only a minor part is utilized. A production plant or an entire company would achieve huge benefits if all this data were combined seamlessly, and the employees would get a total view of the process.
Combining domain expertise with efficient data management
The collected data mass contains a lot of unused business and process improvement potential. To harness this potential, an efficient data handling system is needed.
An efficient data management system enables the combining of all data sources into one system. A user-friendly user interface supports the user in getting useful information and knowledge from that huge amount of data. The user shouldn’t need to spend much time getting data into the data handling system; instead, they should just focus on troubleshooting the problem, increasing efficiency or improving the end-product quality.
Relationships between process phenomena are very complicated, and several measurements affect each other. Therefore, there is often a need to combine original measurements into new and more useful measurements, e.g., to calculate dry solid flow based on original flow and consistency measurements. To enable this type of free data modification, there must be an easy way to add one’s own calculations to the data handling system.
Data analysis process: A system-driven workflow or human-driven mindflow?
Process analysis can be started when all relevant data is available in the data handling system. Picture 1 illustrates the simplified process data analysis or troubleshooting cycle workflow.
The first step is to define the target: what do we need to find out?
The next step is to create a working set of measurements. At this point, combining data from different sources into one system is important. The data connection should be online to avoid laborious and slow data imports into the analysis system. Data should also be close to real-time to enable fast reactions to situations that appear.
Process data always contain some bad values, e.g., huge outlier values, erroneous values, and shutdown data values. This type of bad data affects the analysis results a lot, and therefore, those values must be removed before analysis. To perform this kind of data cleansing efficiently, the tool should support the user’s process knowledge. An experienced employee with process knowledge is the best at judging which values are irrelevant, and then those values should be able to be removed easily from the dataset.
When data is cleansed, the user can perform process analysis, e.g., statistics and correlation calculations. In this step, it’s typical that the user already finds some useful information from the data, but when there are many measurements, this can be laborious. Computer power can be used to make this step much more efficient. A computer can quickly check many process and quality measurements to determine which correlates best with the set target. The system should be able to rank the measurements and give a candidate list of measurements that seem to affect the most to the target measurement. In this step, the data is turned into information.
It’s important to remember that the user must have good process knowledge to get useful study results and make reliable conclusions. In this step, the information is turned into new knowledge. If needed, the user can redefine the task and start the loop again.
In real life, a good and efficient data-analysis workflow is not linear and simple as described above; it looks more like a spaghetti ball.
New information and insights inevitably emerge when data is processed through creative cleansing, refining, calculations, and analysis methods. This guides data analysis forward. Often, these new insights result in redefining the original question.
In an efficient data analysis workflow, new insights are created in all steps. Data analysis systems should support this agile and creative work style. Users must be able to modify the dataset, try different data cleansing scenarios, and try different analysis methods agilely.
Better use of data to address productivity and sustainability challenges
While the amount of data is increasing at an accelerating speed, the resources in the mills are decreasing. The competition between companies and mills is tough, and the situation will not be easier in the future. In addition to all this, the importance of saving natural resources is also rising.
This forces companies to run processes more efficiently and sustainably. One of the most cost-efficient ways to achieve the targets is to utilize existing data more efficiently.
Nowadays, mill personnel are required to handle many different tasks simultaneously. This means that they can’t spend much time on data management. An efficient and intuitive tool is needed to combine all relevant data in the same view. The user with good process knowledge must be able to get answers from the system to process problem questions quickly and easily.
Want more insights?
Our sales team is full of data analysis experts ready to help you. Learn more about Wedge process data analysis tool.